Is Reducing False Positive AML Alerts Even Possible?
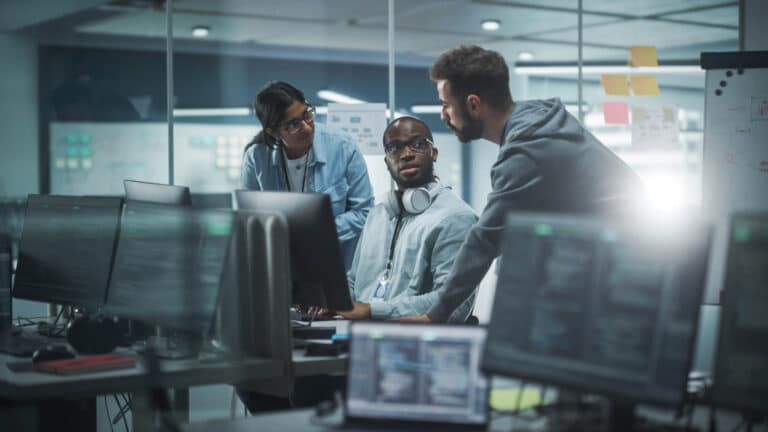
As many as 95% of AML transaction monitoring alerts are false positives, costing billions in wasted investigation time, according to a 2020 report by Global Investigations Review.1
This isn’t a one-off claim.2
Efficient alert resolution remains a top-identified challenge for financial crime compliance operations,3 and the transaction monitoring market is projected to grow from $14.7B in 2022 to $39.5B by 2032.4
Financial institutions understand the problem and seem willing to invest in solutions.
However, the situation remains unchanged.
This article looks at why anti-money laundering (AML) false positives persist, what options exist to substantially reduce false positives, and how we think the market will evolve over the coming decade.
Executive summary:
- False negative rates expose an underlying issue preventing false positive reductions.
- Financial institutions currently rely heavily on open sourced data and manual review processes to identify false positives.
- Creating an automated AML alert prioritisation process that pulls from these same data sources will reduce the negative impact of high false positive rates generated by transaction monitoring systems (TMS).
Suggested reading: To learn more cutting-edge AML tactics, download our free guide — How Leading FIUs Are Using OSINT.
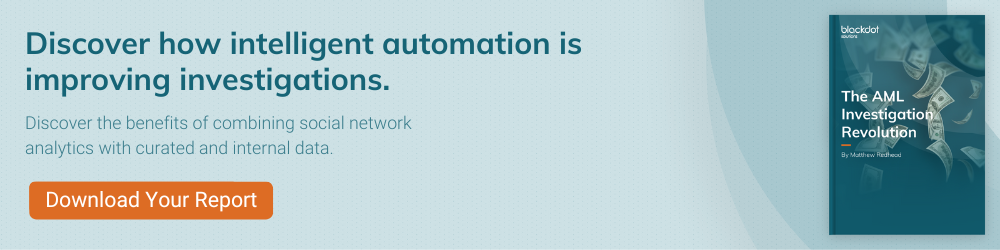
Why AML false positives are so persistent
“The challenge is access to information, and not just about the transaction — information about the people and businesses involved.” — Charles Brown, Head of Community at Blackdot
Of the estimated $800B in money laundered each year, a figure that could be closer to 2T, only about 1% is intercepted by the authorities.5 The unfortunate reality is that even with a 90%+ false positive rate, the false negative rate is likely higher.
When researching how to reduce AML false positives, advice generally centres on taking a risk-based approach, as recommended by FATF.6 Identifying high risk and low risk transactions is a critical part of the process. However, with false negatives so high, it’s likely not possible to substantially reduce false positive rates without a larger change.
The problem, fundamentally, is information access. Transaction monitoring systems (TMS) often don’t have access to the data they need to reliably identify suspicious transactions and money laundering activity. Information uncertainty cannot be entirely removed from this process for three main reasons:
- Uncertainty about external parties: A financial institution’s customer will have been through a KYC (know your customer) process. But there is often less overall information available about external parties via traditional data sources. Business transactions create even more uncertainty because of the challenge to identify the Ultimate Beneficial Owner (UBO).
- KYC and Due Diligence limitations: Although financial institutions are in control of their own KYC and onboarding due diligence process, there are competing priorities when it comes to the speed of onboarding and the level of scrutiny applied. A reasonable motivation to bring on customers quickly can limit the information available for transaction monitoring purposes.
- Time lags: Customer risk profiles, AML risk indicators, transaction scenario parameters and more all need to be updated regularly. Failure to do so was a primary contributing factor to HSBC’s £63.9M fine in 2021.7 However, even compliant operations leave lags between updates, introducing further gaps into the information available to AML programs.
Transaction monitoring processes must walk the fine line between being secure while keeping customer operations efficient. For transaction monitoring systems to be effective at all, we should expect high volumes of false positives. Basic alert scoring is not enough, however. Institutions need to focus on making sure that their screening processes involves as much relevant data as possible, otherwise their alerts can become as much a hindrance as a help.
What’s missing from current transaction monitoring systems
“Transaction monitoring systems have to cast a wide net. The problem is what banks do with those alerts next.” — Charles Brown, Head of Community at Blackdot
Considering the number of false negatives, the volume of alerts raised by transaction monitoring systems isn’t the real problem. What’s missing from this process is the automated capture and analysis of additional open source data to reduce the number of cases that teams need to manually review.
Financial institutions already spend over $54B a year on transaction monitoring operations, 60%-80% of which is labour costs.8 This is indicative of the solution most financial institutions have pursued: throw people at the problem.
If you can’t reliably reduce automated false positives, you can pay people to review the alerts generated. This opens up financial institutions to compliance risk if the volume of alerts surpasses their realistic ability to resolve those cases in a timely manner, or if inconsistencies exist in how those cases are reviewed. Assigning more people to AML departments does not therefore solve the problem of abundant false positives. Instead, FIs should look to intelligence-led automation platforms that utilise or combine transaction data with additional, richer data sets, so that every manual review that is called for is worth the time.
Defining the critical parts of this manual review process is central to identifying how it can be automated. From this perspective, there are three main tasks:
- Identity verification and disambiguation: Particularly when it comes to external parties, it can be difficult to identify exactly who they are. For example, there are nearly five thousand John Smith’s in the UK,9 and a combination of cross-referencing public and private data sources is required to ensure a financial institution knows which one, specifically, is involved in a transaction.
- Negative news screening: Many search engines host huge quantities of personal information that isn’t captured in financial records, peps and sanctions lists, or corporate data. A large part of manually reviewing alerts involves screening news stories to identify potential bad actors. Finding relevant news is a huge challenge. So too, again, is identity verification within this process.
- Connection mapping: With additional information, teams then map connections to identify suspicious behaviour. This is particularly critical for counteracting the use of “mules” — third parties used to mask criminal activities. Visualisation can be enhanced with the use of specific tools.
False positives are primarily identified by gathering additional information, generally relying on open source internet data to crossmatch and add missing details. Although still not a requirement itself, AML compliance trends (e.g. 6AMLD and the AML Act of 2020) have implied the importance of accessing open source data (including adverse media) by stressing the need to act on all readily evident risks.10
Financial institutions need AML alert prioritisation processes that rely on high quality data sets more than they need to reduce the number of false positives initially generated by transaction monitoring systems.
How transaction monitoring can reform
A TMS is more than capable of screening the size, frequency and nature of transactions in order to spot unusual behaviour. However, when it comes to contextualising this data based on the risk profile of both the initiating and recipient parties, they are limited by the scope and sophistication of those profiles.
Financial institutions need more efficient ways of understanding who they are doing business with, and the ability to keep that information up to date. What’s more, they need a way to do this without replacing or undermining the functional systems they already have in place.
There are three components that, when combined, can transform this process:
- Open source data: OSD, or internet data, covers all publicly available information. As discussed, open source data is a large part of the manual review process. This information is already key to identifying false positives. Automating how it’s reviewed will accelerate that discovery.
- AI and IA: Artificial intelligence, and intelligent automation, are two sides of how to apply technology to the transaction monitoring challenge. AI systems learn based on how they perform. This type of adaptive technology is already improving the results delivered. IA keeps humans in the loop and flags low-confidence decisions for manual review. Critically, however, it does this with full context. Technology that’s able to make more decisions and provide more context for human approval when needed will accelerate and simplify manual review processes.
- Modular technology: One tool cannot perform every task. Specialisation is a broader software trend, impacting many types of AML software. What financial institutions need is technology that’s able to work with their existing transaction monitoring systems in order to automate significant portions of the review process applied to generated alerts.
Large numbers of false positives are only a problem without an efficient way of screening those alerts. Financial institutions need to adopt technologies that automatically assess the right kinds of data and then prioritise alerts based on the resulting evidence. This more optimised style of process would further improve the consistency of reviews and investigations.
Financial institutions need an AML alert prioritisation system that better utilises higher quality data to sit alongside their TMS.
Information access and automation are key
Financial institutions spend an estimated $274.1B on financial crime compliance, and 63% claim that AFC compliance additionally negatively impacts productivity and customer acquisition.11
Compliance, business growth and operational efficiency will always cause friction. However, by automating the analysis of wider data sources, including open source data, financial institutions can:
- Reduce the financial burden of compliance, particularly when it comes to resolving false positive AML alerts.
- Remove risks associated with manual and inconsistent data collection.
- Reduce the risk of collecting data and not acting upon it.
- Spend more time investigating money laundering activity and filing detailed suspicious activity reports (SAR).
- Stop more financial crime.
Financial institutions are already doing the right things. They just need to improve the efficiency of their procedures to better combat the challenges they face. Transaction monitoring false positive rates are just one symptom of a wider issue.
Helping financial crime investigators use the right data sets is exactly the problem we’ve set out to resolve at Blackdot. We’ve spent the last decade working with banks, government intelligence agencies, law enforcement and private investigators to apply and build technology able to assess more accurate and relevant data. Blackdot’s technology uses OSD to achieve the breadth and depth of data needed to drive intelligence-led automation.
Our Open Source Intelligence (OSINT) platform is able to automate information gathering, cross-referencing and report visualisation to improve investigations and accelerate decision-making. This technology can integrate directly with your TMS to provide a second layer of automated screening, and dramatically reduce the number of false positives requiring manual review. It’s already played a significant role in counter-terrorism and organised crime investigations, and is being piloted by financial investigators at Danske Bank.
Get in touch if you want to learn more about how technology and OSINT can reduce false positive alerts and deliver a step change in AML investigations.
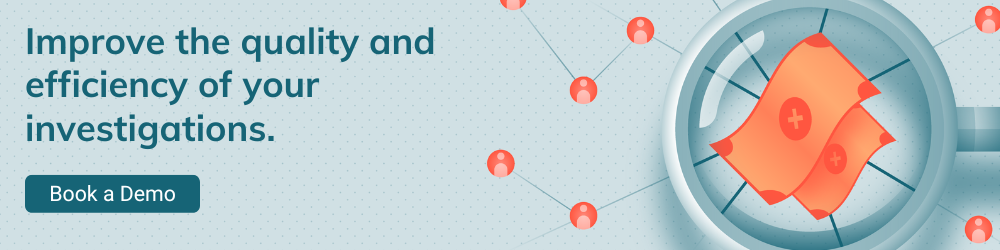
1 Anti-Money Laundering Trends and Challenges – Lexology
2 Anti-money laundering controls failing to detect terrorists, cartels, and sanctioned states | Reuters; The Future of Transaction Monitoring: Better ways to detect and disrupt financial crime | The SWIFT Institute
3 True Cost Financial Crime Compliance 2022 | LexisNexis Risk Solutions
4 Transaction Monitoring Market Size, Share Report 2032
6 FATF: Risk-Based Approach Guidance for the Banking Sector
7 FCA fines HSBC Bank plc £63.9 million for deficient transaction monitoring controls
9 How common is my name? | UK’s most common names revealed | GBG
10 Lex.europa.eu_6AMLD; The Anti-Money Laundering Act of 2020 | FinCEN.gov
11 True Cost Financial Crime Compliance 2022 | LexisNexis Risk Solutions