What is Investigative Screening?

By Rebecca Lindley
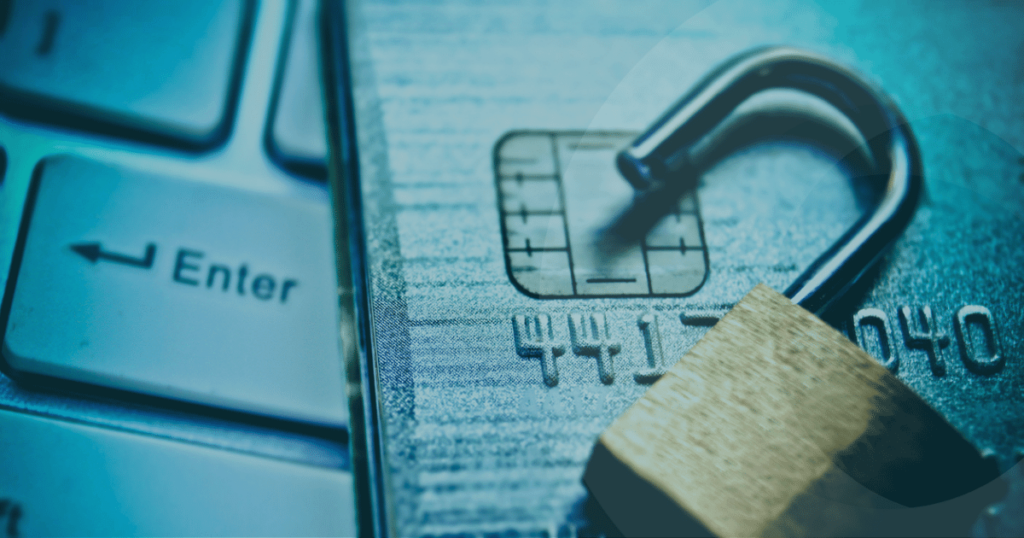
Get the latest news and insights sent straight to your inbox
A new approach to adverse media in effective financial crime risk management
Adverse media is used across numerous functions in financial institutions: from onboarding checks, to transaction alert checks, to enhanced due diligence. Yet its prevalence as a topic across events, webinars and literature underlines the extent to which effectively leveraging adverse media within anti-financial crime processes is a challenge for financial institutions – and that the majority of FIs have not yet found a solution that truly works.
Currently, analysts rely on curated databases to identify adverse media, but these have clear limitations and provide a limited view of risk. With no way of disambiguating data relating to common names and no data on an individual’s associates, analysts using databases of adverse media articles can rarely be certain that they are combatting financial crime effectively. When analysts do use external data to identify adverse media, it is often accessed through search engines such as Google, which are not designed for professional analysts and do not always show the most relevant data.
This is where Investigative Screening comes in. By combining internal and curated data with selected external data sources, and utilising network visualisation, analysts can conduct adverse media checks in a new way that balances thoroughness with speed.
In this article, we’ll examine the challenges that have led adverse media to become such a significant topic and explore how Investigative Screening can augment the benefits of adverse media screening and other manual approaches to external data.
The data problem
The data that is currently used by analysts to conduct adverse media checks is incomplete and unreliable. Firstly, analysts are overly reliant on relatively static databases. Although these databases are updated, their contents will never be as current as the live internet, meaning that it’s difficult to be certain that data is correct. What’s more, in many cases analysts require context beyond that which is available in these databases to allow for effective disambiguation. For example, a common name may appear multiple times in a database, but analysts need additional context in order to understand whether the individual in the database is the same as the person who has been flagged.
Checking associates is also an increasingly important topic due to the widespread sanctions now imposed on Russia-connected individuals and entities. Many regulators require financial institutions to check whether known associates of a client are sanctioned. As databases do not provide detailed information about an individual’s network, other data sources must be used to fulfil these regulatory requirements.
When analysts do need more detail about adverse media, they may turn to the internet and manual Google searching. Naturally, search engines provide access to a multitude of data, but this creates a new problem for analysts: they must scan large volumes of data with no guarantee that they have reviewed the most relevant or important sources. Standard search engines are optimised for advertisers and consumers, not for analysts . Search engine optimisation (SEO), previous online activity and the right to be forgotten can all conspire to mean that results are inconsistent and hard to find without specialist tools.
The process problem
The combined limitations of curated databases and manual search engine searches are an enormous challenge for analysts. However, this is not the only challenge they are faced with: processes within anti-financial crime teams often create more barriers to effectiveness.
Adverse media checks are generally conducted one by one, with few allowances made for interconnection. Yet financial crimes are often highly interconnected. The current approach means that analysts cannot spot patterns or connections between cases that could save them work in the long run.
Similarly, there is a siloed approach between departments. For example, when a case is escalated to a complex investigations team, limited information is passed on. When a member of the complex investigations team picks up this case, they may then have replicate much of the work that the screening team has already done. This contributes further to lack of efficiency.
In fact, current processes are so predictable that more experienced criminals may know which behaviours will be spotted and employ strategies to avoid them.
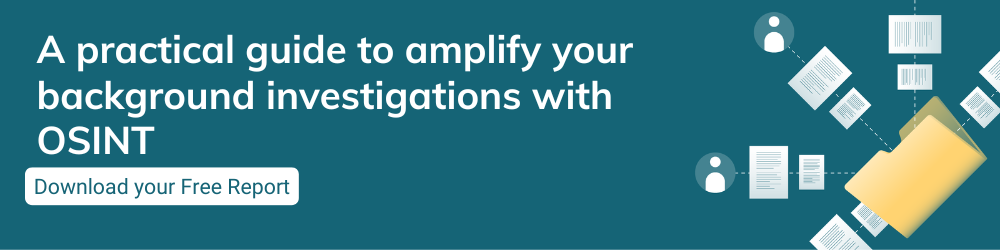
The people or automation problem
In adverse media investigations there is a constant tension between the need for manual, human work and the growing trend for automation. Human judgement is needed for accuracy but taking a entirely manual approach to investigations is slow and can be inconsistent. However, a fully automated approach does not allow for the nuance or context that an analyst needs and produces vast numbers of false positives that then need manual remediation. Many existing approaches are also reliant on curated databases, which brings many of the problems already discussed.
Automation is currently something of a ‘trend’ in financial institutions and is often employed to fix challenges within screening and onboarding, which can result in automation layered on automation. The issue with this approach is that over-automation can often square the volume of false positives that humans are then needed to disposition – what is needed is a considered approach, blending the best of automation technology with tools that truly enhance the analyst’s workflow.
Investigative screening
The challenges we’ve discussed above make it clear that financial institutions need to find a balance between using curated data sources and accessing live, external data more effectively. This needs to be done in a way that allows analysts to spot patterns, find connections between cases and escalate investigations quickly with much-needed context. A balance must also be struck between the efficiency benefits of automation and the need for skilled analysts to make sense of data.
Investigative screening through Videris provides a solution to these issues. This approach draws inspiration from public sector investigations and intelligence methodologies to drive greater efficiency and effectiveness in AML/AFC investigations. In a nutshell, it harnesses adverse media, open source intelligence and network visualisation technologies to ensure alerts can be checked in a manner that balances thoroughness with speed.
Why Videris makes investigative screening more efficient
- Better data – By combining curated data sources with live, external data, Videris allows analysts to access the additional context they need for effective investigations
- Accurate external data – Analysts can automate the collection of external data through a single, secure interface, then filter quickly to the most relevant results. This gives them the confidence that they’ve reviewed all relevant data, without negatively impacting efficiency.
- Slots into existing systems – A case can be auto-populated from a transaction alert system, begun by a screening team, then escalated to a complex investigations team -all within a single, auditable platform and interface.
- Intelligent automation – Videris automates the parts of screening investigations that do not need a skilled human’s input – such as the collection and categorisation of data. Analysts can benefit from efficiency gains without sacrificing accuracy.
- Better people retention – Currently, the Level 1 analyst role is largely a simple exercise in meeting SLAs with limited information to do so. With Videris, this role can become a more productive, information- rich experience, helping staff to become more engaged and feel they are actually fighting financial crime rather than ticking boxes.
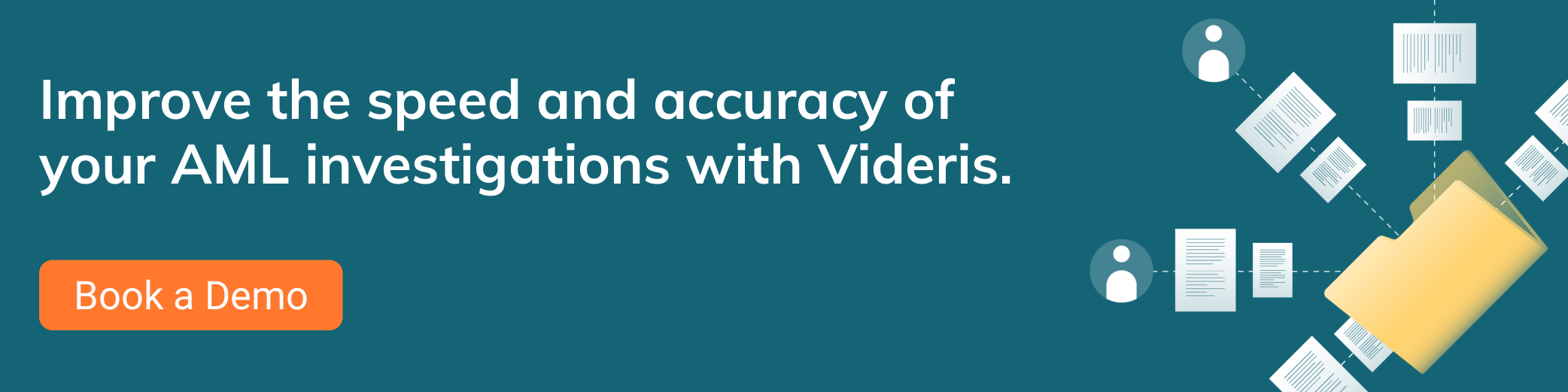